Assessing paropsine damage on Eucalyptus trees with remote sensing
By Leslie Mann, Justin Morgenroth, Cong (Vega) Xu, and Stephen Pawson, November 2022.
Download SWP-T153 (pdf)
Executive summary
New Zealand forests comprise 10.1 million hectares of forests. Due to the sheer scale of managing these forests, remote sensing is increasingly used as a source of information for decision-making. Information on tree growth, mortality, and health related to climate or pest activity can be monitored and quickly mapped. Currently, no remote sensing methods exist to quantify foliar browse by paropsine beetles on Eucalyptus. Currently, defoliation assessments are performed through visual methods by ground-based observers. Such methods, like the Crown Damage Index (CDI), are time- consuming, particularly at larger spatial scales, and potentially suffer from observer bias.
Paropsine damage does not induce a colour change in foliage as would occur with a leaf-sucking insect. Instead, paropsines reduce canopy density by eating parts of leaves, thus altering their shape and area. Hence, LiDAR could be a suitable tool for paropsine defoliation assessment. This study aimed to evaluate the potential for LiDAR as a quantitative assessment of paropsine defoliation of Eucalyptus crowns as a replacement for the CDI.
Three LiDAR scanners (VUX-240, VUX-1LR and L1) were used to collect data from a Eucalyptus trial in the Canterbury region (43°11'47.2"S 172°39'06.1"E) in September 2021 and March 2022. To measure the defoliation prediction accuracy of LiDAR we simultaneously collected CDI data for 55 tree crowns at the same date as the LiDAR data. A total of 57 LiDAR metrics were extracted for each of the 55 tree crowns. The best metrics model to predict CDI was statistically analysed with a Partial Least Squares Regression (PLSR).
Results:
The results demonstrated 18 LiDAR metrics of interest and showed that LiDAR scanners could predict CDI with ±19.1-23.6 % error from the actual CDI observed in the field, with VUX-240 having the smallest error prediction (Root Mean Square Error (RMSE)=9.5 CDI units in September 2021), followed by the L1 scanner (RMSE=10.5 CDI units in March 2022), and VUX-1LR having the highest error prediction (RMSE=11.8 CDI units in September 2021 and RMSE=11.6 CDI units in March 2022).
Key conclusions are:
- All three scanners had comparable predictive abilities, meaning that all could possibly be used for paropsine defoliation assessment.
- The actual error prediction shows promise as a healthy tree could be distinguished from a heavily defoliated tree.
- More testing needs to be undertaken to increase the LiDAR defoliation prediction accuracy. These tests should occur in sites with a broader CDI range (e.g., the Marlborough region).
- Future work needs to move away from the CDI and use a quantitative method of assessing crown defoliation that can be compared with the remotely sensed LiDAR data. This is important as the CDI is semi-quantitative and potentially subject to observer bias.
- More testing needs to be undertaken to determine whether LiDAR can differentiate between paropsine beetle defoliation and trees where abiotic stresses have led to small leaves and/or sparse crowns
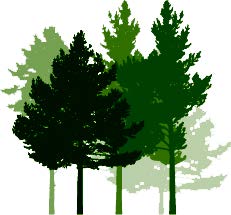
No posts yet
Add a post